I am very excited to share details of our Core AI project which forms the basis of all our solutions and hopefully many more going forward. Recently we released Version 1.3 of this Core project that is named “Doc Element Identifier” or DEI. At a basic level DEI is a pipeline of object detection and block reconciliation deep learning models designed to locate and extract valuable information across a large variety of different document types without the need for a large data set for training.
This article will introduce the problem statement, overview of the solution, discuss results and share improvement we plan in future releases.
But before we jump in, I would like to acknowledge the vision, extraordinary efforts, and the execution of our young talented team. By no means this is complete, we are just warming up.
The Challenge
In the field of Document to Data (or Intelligent Document Processing or Intelligent Data Capture), the purpose of the solution is to take (un)structured data and find meaningful information without having to rely on templates/formats. When we set out to work on this challenge, we kept two goals for ourselves:
1. Obtain a structured output with extremely high accuracy from all kinds of documents.
2. “Zero (near) cost deployment”, which required that the solution should have pre-trained models that can be extended to un-known document types quickly, resulting in timely and cost-effective implementation. Also, for us it will give our operations team a platform that is easy to maintain.
When we initially approached this problem, we started working on use cases such as Identity Cards (for KYC), Receipts, Invoices (for Accounts Payable Automation) and Bank Statements (for Bank Statement Analyzer). However, as we progressed, and encountered multitude of other document types such as “Contracts”, “Financial Statements Analysis”, “Discharge Summaries” etc. we soon realized that “Obtaining Higher Accuracy” and “Zero Cost Deployment” is becoming a trade-off. Individual teams working on diverse problems and trying to achieve higher accuracy, started building their own pipeline of models, which may or may not work for other document types.
Also, AI feeds on the Data for accurate predictions. In our case this data is sample documents and images. With the siloed approach the solution eventually built needed a much higher volume of sample data which was difficult for clients to share (most cases) and stretched the training process.
We needed to go back and think differently.
The Solution
All documents, no matter how diverse, can be broken down into segments OR blobs of text. Simply put these segments can be Header, Footer, Tables, Headline, Sub-Headline, Paragraph and so on. While the document types can be infinite, the types of segments, or elements as we call it, in any document will be finite. What if we break down every page of a document and understand these segments and then look for the data attribute we are finding in that segment?
And this is how we as humans read a document. E.G. After you have eaten at any restaurant globally (I want to harp that its irrespective of the language) and when the check arrives, the very first thing we do (or at-least I do, if I am paying) is to look for the check amount. When we do this, our eyes will automatically go to the end of a Table in the receipt for that “Total Amount” and no-where else. Now if a model can locate a Table in a receipt and decipher its last row, we know where the Total for not just this receipt, but all receipts will mostly be.
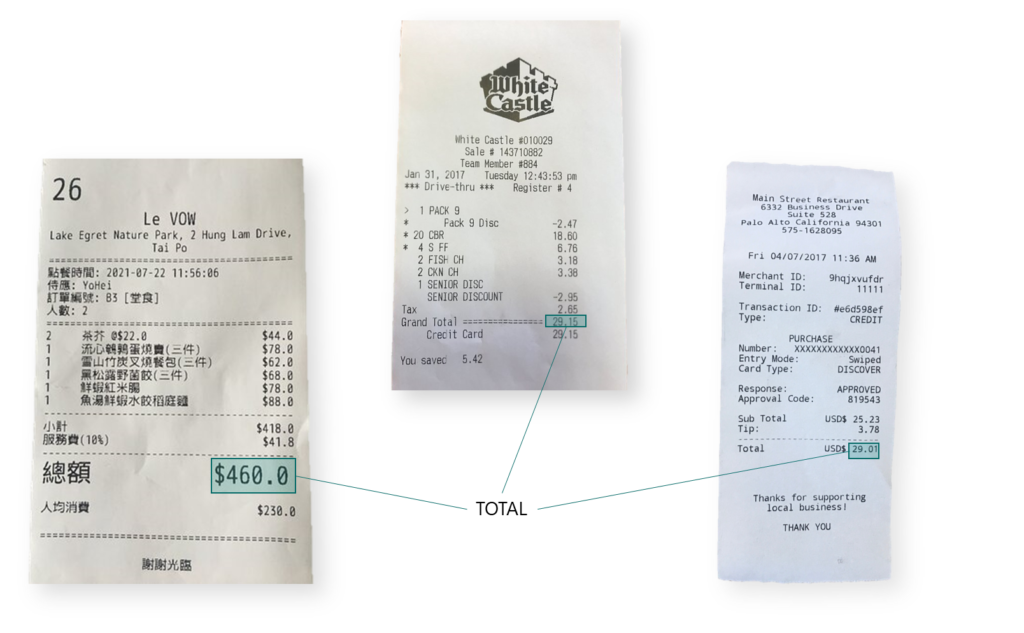
How was the Solution developed?
We built a deep learning-based technique with two diverse objectives
1. Predict different layout elements starts with training multiple layout-detection Deep Learning (DL) models in image domain in a pipeline, followed by different block reconciliation algorithms that rearrange document text detected by an OCR engine into an appropriate layout block.
2. Provide use case agnostic tabular data reconstruction in documents. Table reconstruction relies on table cells to identify table rows and table columns. A ‘table cell’ is the smallest unit of a table formed by combination of rows and columns. A table cell detection module is trained to identify different cells in a table, and these cells are then grouped together in rows and columns fashion by a clustering module.
Identifying different layouts elements in documents is not new, in most of prior technologies it uses non-deep-learning methods e.g., internal structure/s of a table was identified based on different heuristics that involved identifying the vertical and horizontal alignments, so that all OCR text can be placed in a proper row-column arrangement. Although these kinds of heuristics worked well for standard tables structures (with identified and well segregated rows and columns), most of the tables in business documents such as Invoices or Bank Statements fall in exceptional cases, where these prior technologies did not work.
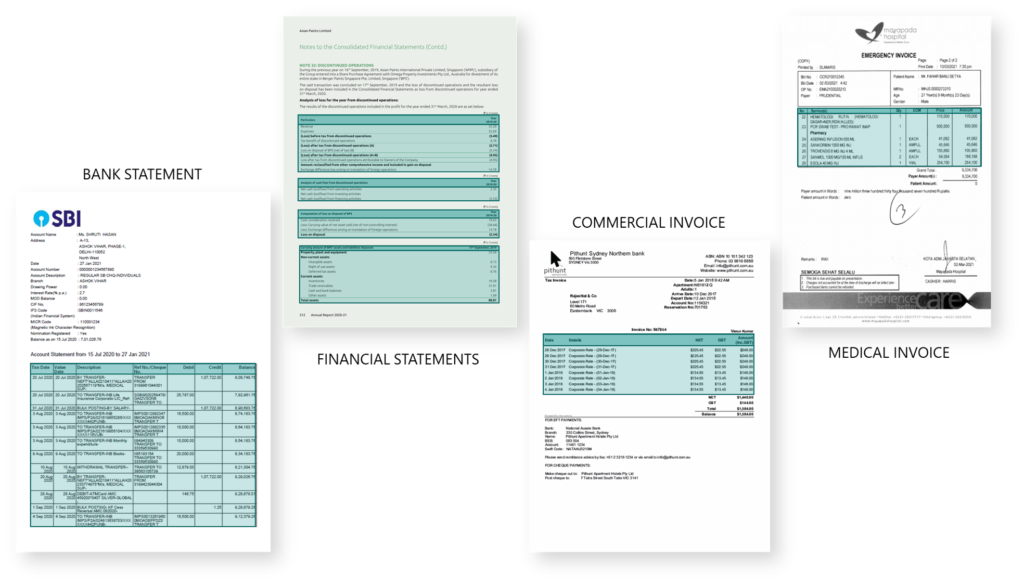
The Result
It took our team a god year to conceive, develop, iterate and improve DEI, and the results are very encouraging:
- Incorporating this core-component as the base layer makes use-case specific and template agnostic attribute extraction from documents a very simple and faster task.
- Focusing on document elements allowed us to train new models for any document type with a much small training dataset.
- Availability of the reconciled table in digitized form from this core component, makes the table line-item extraction a very simple task. This is a huge competitive advantage for us as we can use it for multitude of use cases without needing any training data
- The approach, as it works in the image domain, is largely language agnostic. We have tested the Invoice and Bank Statements models for Malay, Latin and even Arabic documents and the models worked with very limited effect on accuracy.
What’s Next?
We expect this breakthrough technology to bring a paradigm change in the “Document to Data” space. This Core invention gives us the ability to deliver “Highest Day One Accuracy” at “Breakthrough speed”, which is unheard of previously. And we are continuously improving, look out for this space for truly global Universal Document Reader, where we will launch a platform that understands an industry specific domain and vernacular. This completely take away the need to use engineering team to develop new model requiring a data-science project for any use-case.